Noise Reduction Techniques in Podcast Editing
Noise reduction is essential for professional-sounding podcasts. This guide dives deep into the evolution of noise reduction techniques, from traditional methods to cutting-edge AI, and reveals how to chain these processes for truly exceptional results.
Topic | Details |
---|---|
Types of Noise | Static (white, pink, brown), HVAC, electronics hum, transient noises (doorbells, dogs, clicks), sibilance, background conversations |
Traditional Techniques | Spectral Subtraction with Fourier Transforms, Noise Profiles, careful attenuation to avoid artifacts like the "underwater" effect |
Machine Learning Subtractive Tools | RX, Accentize VoiceGate, Izotope RX, Waves Clarity, Acon Digital, Focus on non-stationary, disruptive sounds, require careful preprocessing |
AI-Powered Reconstructive Denoisers | Adobe Enhance, Descript Studio Sound, Accentize DxRevive, Address signal degradation from noise removal, excel with phone recordings |
Best Practices | Quiet recording environment, optimize mic placement and settings, gentle, multi-pass processing, addressing sibilance challenges, consistent noise floor |
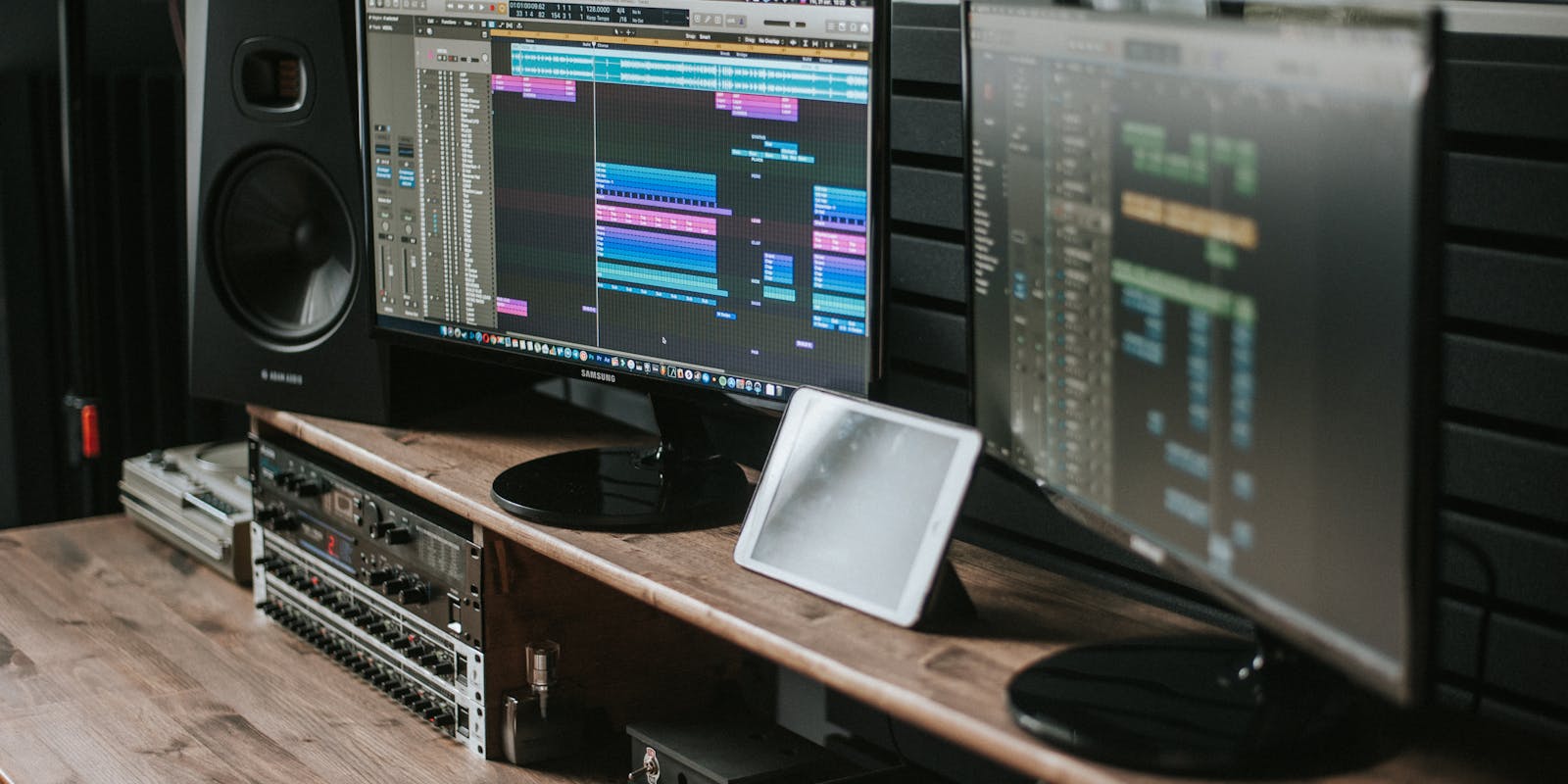
The Evolution of Noise Reduction: From Static to Sibilance
Noise in podcasts comes in many forms, from the constant hum of electronics and HVAC systems to sudden bursts of transient noises like dog barks, door slams, or keyboard clicks. Even sibilance, those harsh "s" sounds, can be a form of noise that requires specialized treatment. Understanding these different types of noise is crucial for selecting the right reduction technique.
Until recently, noise reduction relied on spectral subtraction. This method uses Fourier Transforms to analyze the frequency spectrum of your audio. By creating a noise profile during periods of silence, you could theoretically subtract those frequencies from the overall signal. However, this approach struggles with transient and dynamic noises, often creating a hollow, "underwater" effect if pushed too hard.
Then came machine learning-based subtractive tools. Programs like Izotope RX and Accentize VoiceGate revolutionized noise reduction by training algorithms on vast datasets of noise and clean audio. These tools excelled at identifying and removing non-stationary, disruptive sounds that traditional methods couldn't handle. However, they introduced a new challenge: dependence on training data. If the algorithm wasn't trained on a specific type of noise or voice (like a child’s voice or a very deep voice), results could be unpredictable.
Now, we're in the era of AI-powered reconstructive denoisers. Tools like Adobe Enhance, Descript Studio Sound, and the Emmy award-winning Accentize DxRevive not only remove noise but also attempt to *rebuild* the underlying audio signal that’s often degraded during the noise reduction process. This is particularly effective for cleaning up phone recordings and combating artifacts. However, these tools also benefit from careful preprocessing and a nuanced approach.
Modern Noise Reduction Workflow: Chaining for Superior Results
Modern Noise Reduction Workflow: Chaining for Superior Results
The most effective modern approach involves strategically chaining different noise reduction tools. Instead of relying on one tool to do everything, you create a multi-stage process, each step addressing specific noise types and minimizing artifacts. Here's a typical workflow:
- Preprocessing: Address clicks, pops, and other transient issues using dedicated declickers and declippers.
- Machine Learning Subtractive: Use tools like RX or Accentize VoiceGate on a low setting (10-20%) to tackle broader noise issues and transient sounds without overly impacting the signal.
- Spectral Denoising: Employ a spectral denoiser like NS1, which incorporates a soft gate for consistent noise floor, to further reduce residual static and hum.
- AI-Powered Reconstruction: Finalize with Adobe Enhance, Descript Studio Sound, or DxRevive to address signal degradation, refine the audio, and minimize any remaining artifacts.
This layered approach minimizes the workload on each tool, producing cleaner, more natural results while reducing the risk of unpredictable AI behavior.

Addressing Sibilance and Other Challenges
Addressing Sibilance and Other Challenges
A persistent challenge with machine-learning denoisers is their tendency to misidentify sibilance, especially in higher-pitched voices, as noise. This can lead to a lisp or unnatural thinning of the voice. Addressing this requires a combination of strategies:
- Spectral Denoising First: Applying spectral denoising *before* machine learning subtraction can help mitigate this, as spectral tools are less likely to target transient sibilance.
- Gentle, Multi-pass Processing: Use multiple, light passes with your subtractive tools rather than one heavy pass. This allows greater control and minimizes unintended consequences.
- Dedicated De-essing: In some cases, a separate de-essing plugin might be necessary to tame sibilance after noise reduction.
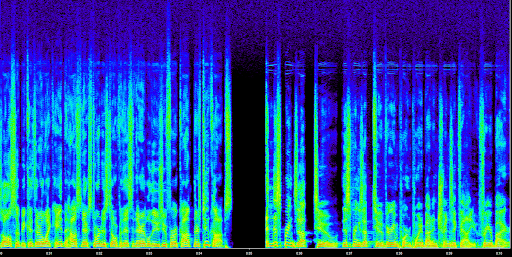
Finding the Right Balance: Avoiding Over-Processing
Finding the Right Balance: Avoiding Over-Processing
The goal isn’t to eliminate every bit of noise, but to create clear, engaging audio. A touch of background ambience can add a sense of realism. Over-processing leads to robotic, unnatural sound.
- Start with minimal settings and incrementally increase only as needed.
- Test your audio on multiple devices to ensure it translates well.
- Listen to professional podcasts in your genre as a benchmark.
- Take frequent breaks to avoid ear fatigue and maintain perspective.

Conclusion: Mastering the Art of Clean Audio
By understanding the different types of noise, selecting the right tools, and implementing a strategic workflow, you can elevate your podcast audio to a professional level. The combination of traditional and AI-powered techniques offers unprecedented control and quality. Remember, the key is not just noise reduction but audio enhancement - creating a listening experience that is both clear and engaging.